Table of Contents
Financial institutions have always relied on data. However, the sheer amount of information they collect today is unprecedented. To stay competitive in the modern market and further grow your business, it is crucial to know how to use these volumes of data to your advantage. And that’s where big data analytics emerges as a game-changer.
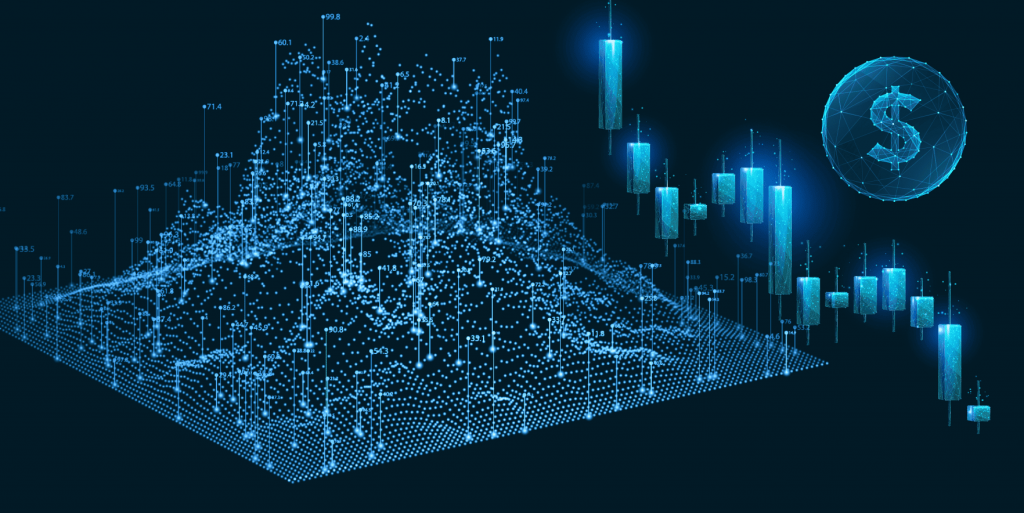
According to Verified Market Reports, the global market for big data analytics in banking is expected to reach $745.16 billion by 2030. Statistics speak louder than words, so there’s no doubt that big data services will continue gaining popularity in finance.
In our article, we will explore applications and advancements of big data analytics in finance, the latest technology trends that shape this rapidly evolving sphere, and the challenges you might face along the way.
The role of big data in finance
Big data analytics is a collection of techniques used to process and analyze vast amounts of information. It incorporates machine learning, predictive analytics, data mining, and natural language processing to identify patterns, relationships, and insights that traditional methods often overlook.
The financial sector is one of the most data-driven industries. Traditionally, analysts performed all calculations and market trend analyses manually. However, with the rise of digital banking, the amount of information the sector generates today is enormous. Data comes from various sources and greatly varies in format. It’s not just transactional records and account statements but also:
- Social media activity;
- Exchange rates;
- Stock prices;
- Demographics;
- Spending habits and preferences;
- Investment activity, etc.
Analyzing such massive datasets using conventional methods is impractical. Given the rapidly changing financial landscape, the ability to process information in real time is not just an advantage but a necessity.
Big data analytics helps financial institutions to process and analyze these large bundles of data in real-time. It allows them to save the competitive edge, enhance operational efficiency and decision-making, and reduce risks.
Key benefits of big data analytics in finance
As we can see, big data analytics offers numerous benefits to the finance industry. Here are some of the main advantages of big data in finance.
Data-driven insights for better investment strategies
One of the great advancements of big data analytics is data-driven insights that help to develop more profitable investment strategies. With the use of machine learning algorithms, financial analysts can process and analyze historical data, market trends, and economic markers at a large scale. Therefore, they can predict stock movements and market direction to help investors make more informed decisions.
Fraud detection and anomaly identification
Financial fraud is a neverending problem. Technological advancements not only drive digitalization and optimize processes but also give scammers advanced tools for attacks.
Big data analytics helps to identify anomalies and suspicious activities, therefore preventing fraud or information theft. Through advanced algorithms and data mining techniques, financial institutions can monitor transactions in real time, flagging irregularities that may indicate fraudulent behavior.
Personalized financial products
The era of one-size-fits-all financial products is fading. With big data analytics, financial institutions can now tailor their offerings to meet the unique needs of individual clients. By analyzing customer data, including spending habits, preferences, and financial history, organizations can develop personalized financial products.
For example, robo-advisors utilize algorithms to create customized investment portfolios based on an individual’s risk tolerance and financial goals. This level of personalization not only enhances customer satisfaction but also fosters stronger client relationships.
Market trend predictions and risk mitigation
Big data analytics not only helps to enhance investment strategies with data-driven insights but also predicts market trends and mitigates possible risks. Financial organizations can use predictive analytics to understand potential market volatility, adjust their strategies, and take necessary steps to minimize risks.
Regulatory compliance monitoring
Trying to keep up with constantly changing government regulations can be quite challenging for financial institutions, considering the amount of data they work with. Big data analytics can help banks and other organizations adhere to important regulations like GDPR, anti-money laundering, etc.
With advanced BI tools, they can analyze large datasets and monitor and track customer transactions in real time, detecting anomalies or potential breaches. Financial organizations can also use this to track changes in regulations to reduce the risk of penalties and reduce compliance costs.
Applications of big data in finance
As a result, big data has become an indispensable tool for financial organizations. Aside from improving efficiency and risk management, there are many other ways to use big data analytics for financial services. Let’s explore some of the most significant applications.
Credit scoring
Traditionally, credit scoring relied heavily on historical financial data and a few standardized metrics. Big data has transformed this process by incorporating a wider array of data points, including social media activity, online behavior, and even mobile phone usage. By analyzing these diverse datasets, financial institutions can create more nuanced credit profiles, allowing them to assess an individual’s creditworthiness more accurately.
For example, companies can use machine learning algorithms to analyze unconventional data, resulting in higher approval rates for credit applicants who may have been overlooked by traditional models.
Fraud detection
Financial organizations work with highly sensitive data, so it’s no surprise that fraud activity is pretty high there. Big data analytics allows banks to store historical information about previous transactions and provides access to real-time data. Machine learning algorithms can analyze this information and identify suspicious activities or unusual customer behavior. Therefore, organizations can mitigate risks and employ strong anti-fraud measures.
For instance, two transactions from one credit card were made simultaneously or within a short time frame in two different cities. The bank can immediately react and inform the client about the unusual activity and security threats. They can even block the transaction and the card as a preventive measure.
Asset and wealth management
Big data is revolutionizing asset and wealth management by providing a more comprehensive view of investment opportunities and risks. Wealth managers can now leverage data analytics to analyze market trends and individual client preferences simultaneously. This approach enables more informed investment strategies.
For example, machine learning algorithms can process and analyze historical data and current market conditions to identify potential investment opportunities that human analysts might overlook.
Customer segmentation
One of the most exciting applications of big data in finance is customer segmentation. Traditional methods of segmentation used to rely on demographic factors like age, income, and location. However, big data enables financial institutions to analyze a multitude of variables, including purchasing behavior, online interactions, and social media activity.
For instance, banks can use data analytics to identify specific groups of customers who may be interested in particular financial products. By examining transaction histories and customer feedback, they can create detailed profiles that allow for more targeted marketing strategies. This not only improves customer satisfaction but also boosts conversion rates.
Operational efficiency
The financial sector is notorious for its complex and often cumbersome processes. Big data analytics significantly enhances operational efficiency by automating processes, identifying inefficiencies, and streamlining workflows. For example, they can use predictive analytics to forecast cash flow needs, enabling institutions to manage liquidity more effectively.
Technologies driving big data analytics in finance
AI and machine learning
Obviously, artificial intelligence and machine learning are the main driving forces behind the advancements of big data analytics in finance. They enable financial institutions to process and analyze massive datasets with remarkable speed and precision, uncovering patterns and insights that would otherwise go unnoticed.
AI and ML facilitate a wide range of applications in the financial sector, such as:
- Predictive Analytics;
- Fraud Detection;
- Customer Personalization;
- Risk Assessment, etc;
Importance of cloud computing in handling financial data
Cloud computing allows financial organizations to manage and safely store their massive datasets. One of its primary advantages is scalability. Financial institutions can access virtually limitless storage, which allows them to handle fluctuating data volumes without investing in on-premise infrastructure. This flexibility is particularly beneficial when data demands can increase during market volatility.
Transitioning to the cloud also offers cost savings. Traditional data centers require substantial investments in hardware and maintenance. In contrast, cloud services operate on a pay-as-you-go model, allowing firms to allocate resources more effectively.
An overview of big data analytics tools
Given the volumes of data nowadays, it is nearly impossible to process all this information manually. Real-time insights are crucial for organizations to stay competitive and make faster, more informed decisions. Luckily, numerous big data analytics tools are available on the market, so you can choose one that best suits your needs, goals, and budget. However, picking the right one can be pretty frustrating with so many options. Here are some of the key functions that you should look for in an analytics tool:
- The ability to handle large volumes of data. If the tool you choose cannot process vast amounts of data, then there’s no point in using it. Look for high-performance computing capabilities that can scale with your business as data volumes grow without sacrificing performance.
- Data visualization capabilities. Our brain perceives visual information better than text. Graphs, charts, or dashboards will be easier to understand, even for nontech personnel. Make sure you choose the tool with data visualization functionality so you can present the data in a more digestible and actionable way.
- Intuitive interface and ease of use. It’s better to choose a tool with a user-friendly interface and intuitive features like customizable dashboards, drag-and-drop options, and clear reporting. Therefore, it will be easier for your team to master and use the tool efficiently.
- Seamless integration and compatibility with existing infrastructure. Ensure that the analytics tool can integrate seamlessly with your existing systems and tools, such as CRM platforms and cloud and data storage solutions. It’s also essential that the tool can connect and derive data from various data sources.
- Robust security features. With the increasing risk of data breaches, it is critical to choose a tool that offers robust security features, including encryption, access controls, and compliance with industry regulations to protect your data.
- Cost efficient. Define what your needs are and set the budget accordingly. This way, you will know what price options you are looking for. There are a lot of affordable tools with free or low-cost plans, but their functionality can not be enough for you. Therefore, carefully check out the pricing structure of each tool you consider.
- Collaboration features. You may need to share your insights with stakeholders or have different teams working on the analysis and report. So, it’s better if the tool has the ability to share the reports, dashboards, or data sets in real time.
Here are some of the popular tool choices for big data analytics in finance that you can consider:
Apache Spark
Apache Spark is an open-source analytics engine recognized for its speed and ease of use. It offers in-memory data processing, which significantly enhances performance. Additionally, the platform includes several libraries to support SQL queries and provides user-friendly APIs that simplify data retrieval.
MongoDB
MongoDB is a NoSQL database, so it can handle large volumes of unstructured or semi-structured data. It is highly scalable, flexible, and categorizes documents into collections with key-value pairs. Due to its functionality, MongoDB is an excellent tool for content management and real-time analytics. It is also popular among developers because it supports multiple programming languages, including Python, Ruby, and JavaScript.
BigQuery
BigQuery is a managed serverless data warehouse provided by Google Cloud. With its robust built-in features like machine learning, business intelligence, and geospatial analysis, the platform helps users process and analyze vast datasets. BigQuery is highly scalable and cost-efficient; its pricing is based on the amount of processed data or a flat-rate option for predictable costs.
Snowflake
Snowflake is a cloud-based data warehouse that provides flexible and secure storage for large amounts of information. The platform separates storage and compute, allowing users to scale resources independently based on their needs. Snowflake uses a custom architecture that combines shared-storage and shared-nothing system approaches. This means all data is stored in one place and accessible to all compute nodes, but each node processes data independently. Overall, Snowflake is easy to use and also provides multi-cloud support.
Power BI
Power BI is a powerful business analytics tool with robust capabilities that helps transform raw data into interactive visualizations. It seamlessly integrates with other Microsoft services like Azure or Office 365, as well as with various data sources, such as Excel, cloud services, and SQL databases, fostering collaboration and real-time data analysis. PowerBI has a user-friendly interface and is easy to use for non-technical users.
Tableau
Another great option of robust data visualization and big data analytics tools is Tableau. It empowers businesses to interpret and transform raw data into easy-to-understand visualizations. Tableau has a drag-and-drop functionality, which makes it accessible for users with different technical skill levels. It allows users to conduct real-time data analysis and create compelling, shareable dashboards. Overall, Tableau is a great tool for business intelligence and collaborative work.
If you are interested in Tableau and Power BI but don’t know which one to choose, you can check our Power BI vs Tableau comparison article.
Expert Opinion
Big data analytics isn’t just a trend in finance – it’s a must-have for any institution that wants to keep up. With markets moving faster than ever, banks and investment firms need real-time insights to make smart decisions, manage risks, and give customers more personalized services. AI and predictive analytics are already changing the game, helping innovative firms spot trends before they happen. The ones that embrace these tools now won’t just survive – they’ll lead the future of finance.
Challenges of big data analytics in finance
Although big data analytics brings a lot of benefits for the financial sector, providing valuable insights into consumer behavior, enhancing risk management and fraud detection, there are several challenges that companies may face. Let’s explore some of these issues in detail.
Data security and privacy
In the finance industry, the stakes are incredibly high when it comes to data security. Financial organizations handle vast amounts of sensitive information, from personal identification details to transaction histories. Therefore, they need to ensure robust security measures while maintaining user privacy. A breach not only compromises customer trust but can also lead to significant financial losses.
Regulatory and compliance issues
As we already mentioned, the financial sector is one of the most regulated industries globally, with stringent rules and guidelines designed to protect consumers, maintain market integrity, and prevent systemic risks.
Ensuring compliance with evolving regulations requires robust data governance frameworks. These frameworks need to encompass data quality, lineage, and accessibility. As financial institutions collect and analyze data from various sources, maintaining a clear understanding of data origins and transformations can be challenging.
Integration complexity
Many banks and financial institutions still rely on legacy systems that lack the flexibility for sophisticated analytics. Integrating these systems with new technologies, such as cloud computing and machine learning algorithms, can lead to operational inefficiencies. Companies must ensure seamless data flow between systems while maintaining data integrity, which often requires substantial time and resources.
Skill gap
Even though the importance of big data analytics is quite evident, the financial sector is in dire need of skilled specialists. The thing is, data science is a difficult field, and data analysts need a particular set of skills, such as proficiency in programming, statistical analysis, and data visualization. Data analysts must also be adept at working with various data management tools.
An additional complication is that financial institutions require specialists who possess not only technical skills but also understand the complexities of the finance sector, such as market trends, regulatory rules, risk management, and investment strategies. As a result, it’s quite a challenge to find and hire a good specialist.
Data quality
If the quality of your data is poor, so are the insights you retrieve from it. As we mentioned earlier, the sources of financial data, as well as its formats, are quite diverse. Up to 90% of this data is unstructured and difficult to analyze, which can lead to misguided business decisions. So, maintaining the high quality and consistency of the information across all these sources is a critical concern.
Future trends in big data analytics in finance
The financial sector continues to embrace digital transformation, constantly finding new ways to use big data analytics for financial services. And given the technological advancements that have surfaced in recent years, the future looks promising. Here are some trends we can expect moving forward:
Role of blockchain in financial data management
Blockchain technology has great potential in big data analytics. It ensures transparency of financial operations, allowing all parties of financial transactions to view the same data. The decentralized nature of blockchain paired with predictive analytics can help to enhance security measures and fraud detection. Not to mention, blockchain employs cryptographic techniques to secure data, making it resistant to tampering and unauthorized access.
Moreover, blockchain technology supports the use of smart contracts — self-executing contracts with the terms of the agreement directly written into code. These contracts automate processes and reduce the need for intermediaries, streamlining operations.
Expansion of AI-powered tools
Financial organizations are increasingly adopting AI-powered tools to analyze vast datasets, uncover patterns, and make data-driven decisions. Machine learning algorithms can predict market trends, assess credit risk, and even enhance customer service through chatbots.
Moreover, AI’s ability to process unstructured data, such as social media sentiment or news articles, enables financial analysts to gain insights that were previously difficult to capture. And it will only improve from there, as AI and machine learning are constantly evolving. Companies like BlackRock are already leveraging AI to optimize investment strategies, demonstrating the immense potential of these technologies.
Increasing importance of ESG (Environmental, Social, Governance) data analytics
As sustainability becomes a pressing global concern, the importance of ESG data analytics in finance is on the rise. Investors are increasingly considering ESG factors when making investment decisions, leading to the development of tools that analyze corporate performance based on these criteria.
Big data analytics enables financial institutions to evaluate ESG risks and opportunities more effectively. For example, companies can use data analytics to assess their carbon footprint and analyze supply chain practices and employee diversity. With this information, they can align their strategies, making them more sustainable and appealing to investment.
Conclusion
Big data is greatly reshaping the landscape of various industries, and the financial sector is not an exception. In the conditions of high competition in the market, many companies are adopting big data analytics to not only maintain a competitive edge but also evolve their business. Complex algorithms of machine learning models quickly process and analyze all sorts of data, providing valuable insights that help financial institutions enhance their decision-making, plan strategies, and evaluate operational efficiency.
As data technology further advances, companies can create more innovative products and services that meet the changing needs of consumers and investors. However, finding skilled data science specialists for the task can be quite challenging. Therefore, you can always collaborate with experienced big data service providers such as SoftTeco. With extensive industry expertise and technical skills, we can help you leverage the full potential of your big data.
Comments