Table of Contents
One of the most popular technologies in the healthcare sector today is machine learning. Numerous studies emphasize its exponential growth, with the global AI in healthcare market estimated at USD 22.45 billion in 2023 and expected to have a CAGR of 36.4% between 2024 and 2030 (according to the report by the Grand View Research).
The primary reasons for such popularity are that machine learning significantly optimizes and improves patient care while also assisting medical professionals in their work. In this article, we will look at the biggest use cases of machine learning in healthcare and the process of implementing this technology in one’s healthcare digital environment.
The growing role of machine learning in healthcare
The healthcare industry is an incredibly complex one and involves daily processing of massive volumes of clinical data. Hence, healthcare professionals are very open for adcanced technologies that can help in decision making and diagnostics as well as in optimizing certain processes.
The current challenges that the industry faces explain the growing role of machine learning in healthcare and its adoption across medical facilities. These challenges include the growing demand for qualified personnel, too lengthy drug discovery, or inability to recognize certain diseases at early stages. In the light of this, here are some numbers that clearly show how machine learning has become an integral part of healthcare:
- The global generative AI market in healthcare is expected to register the CAGR of 37% between 2023 and 2032 (market.us);
- 36% of healthcare organizations implemented NLP (natural language processing) software;
- Artificial Intelligence in drug discovery market is expected to reach $12.8B by 2032.
The best part about adopting machine learning for healthcare is that technology keeps evolving. That means in the future, its work will be even more accurate and efficient, further transforming the industry for the best.
Basic ML models used in healthcare
When discussing machine learning in healthcare, it’s also worth mentioning the types of ML learning models that can be used. There are three types of models that ML development offers, each with its own machine learning algorithms:
- Supervised learning
- Unsupervised learning
- Reinforcement learning
Let’s briefly look at each.
Supervised machine learning
This learning model is probably the most popular one and is used in wide variety of applications. Supervised machine learning means that the model is trained on a data set where each input already has a label. In this way, the model “learns” on the provided information and uses it to make assumptions about new inputs. An example would be a data set with images of bananas and apples, labelled “banana” or “apple” correspondingly. The main learning algorithms involved in supervised learning include linear regression, logistic regression, random forest, and support vector machines.
In healthcare, this model is used for medical diagnosis as it can learn on existing medical images and assist doctors in disease identification on new images.
Unsupervised learning
While in supervised learning the model learns on already labeled inputs, in unsupervised learning, the model receives a data set without any labels. In this way, the main goal of the model is to independently find patterns and structure within the given data and categorize them correspondingly. As a result, the model groups together inputs with similar characteristics like age, date of birth, location, etc. examples of learning algorithms for unsupervised learning are principal component analysis and K-means.
In healthcare, unsupervised learning usually serves research purposes and is often used in drug discovery, identification of new disease causes or new groups of patients, thus performing clustering.
Reinforcement learning
The third learning model operates by the following algorithm: it performs an action and receives feedback on the performance. Based on this feedback, the model will adjust its future actions to achieve the needed results and improve the performance. In simple words, reinforcement learning means learning from the consequences of the model’s actions. As for the learning algorithms of this model, they include SARSA, A3C, and Q-Learning.
One of the most popular examples of reinforcement learning in healthcare is creation of personalized treatment plans. Based on the patient’s response and the medical history, the model will be adjusting the proposed plan to fully meet the patient’s needs.
Main benefits of machine learning in healthcare
Before disccussing the real-life examples of ML in healthcare, let’s briefly overview the biggest benefits of machine learning for medics and patients:
- Automation of tasks: implementation of machine learning can automate a number of routine tasks and processes, especially in the field of hospital management. Examples include automated collection of clinical data or automated staff scheduling.
- Improved patient care: machine learning is the cornerstone of precision medicine, aimed at delivering personalized care to every patient through careful analysis of their electronic health records, medical history, and genetic profile. Also, ML contributes to more accurate diagnosis making and more effective medical image analysis.
- Extended access to medical care: due to machine learning, patients can now receive remote medical care and use virtual nurses. This greatly increases availability of medical care for a bigger number of patients.
- Better risk prediction: the ability of ML models to identify unobvious symptoms and patterns in one’s medical history plays a big role in disease risk prediction and prevention. As well, ML is the cornerstone of predictive analytics, which helps with forecasting the probability of disease development and its severity.
Key machine learning use cases in healthcare
Implementation of the ML technology in healthcare facilities is beneficial for both patients and doctors on multiple levels. Below, we look at the most prominent machine learning use cases and real-life examples of machine learning in the industry.
Disease prediction and diagnosis
Accurate disease prediction remains one of the biggest challenges in healthcare, and machine learning is used to facilitate and automate the process to a certain extent. ML-based image recognition is one of the most common use cases of this technology in diagnostics since the ML model can recognize and detect patterns that were previously unknown or unnoticed by medics. This, in turn, allows early detection of diseases and their timely prevention, increasing the chances for successful treatment.
Also, don’t forget about predictive analytics that can be used to forecast the potential disease development or future chances for disease outbreak. Such forecasts are created based on the historical data of the patient combined with the newly collected one and significantly help doctors in analyzing the disease flow and the possibility of its development.
Medical imaging
Medical images include X-ray scans, MT, and MRI scans and help medical professionals detect diseases at various stages of development. Unfortunately, medical imaging depends heavily on one’s ability to analyze these images properly, and hence, the chance of a human error remains quite high.
The use of machine learning in medical imaging eliminates the possibility of a human error and can detect even the slighest alterations from the norm, thus allowing doctors to faster detect anomalies and prevent illnesses from progressing.
Personalized treatment
Personalized medicine, also known as precision medicine, is on the rise these days due to the advancement of machine learning. This practice is aimed at closely studying one’s genetic profile and health records in order to select the most suitable treatment and to accurtely predict how a specific patient will respond to it. This approach to patient care significantly increases the chances of a patient for positive immune response and successful outcome of the treatment. In this use case, machine learning helps medics quicky and effectively analyze the patient’s data and detect which drugs and treatment plans would be the most compatible.
Drug development and discovery
Another big challenge of the healthcare industry is the process of drug discovery and development. First, it is very expensive, and second, it might take years to develop a single drug due to lengthy clinical trials. Machine learning greatly assists here by speeding up the process of finding combinations of components and drawing accurate predictions on how a specific drug will perform. Doctors can also use ML to identify new use cases for already existing drugs, thus expanding the area of their use.
Virtual nursing
The outbreak of COVID-19 served as a catalyst for many changes in the healthcare system, with virtual nurses probably being among the most prominent ones. A virtual nurse is an ML-based personal assistant that serves the following functions:
- Sends reminders and notifications about upcoming appointments and the need for their scheduling
- Helps in performing regular health checkups
- Sends reminders about the need to take medications
- Connects a patient with their doctor
- Monitors electronic health records of a patient
- Sends health alerts
Usually, a virtual nurse is an app with an AI bot that communicates with the patient, analyzes their medical history, and provides valuable sugestions according to their specific case. The biggest benefit of virtual nurses is their 24/7 availability and immediate response.
RPA in surgery
Robotic process automation (RPA) powered by machine learning is a powerful tool that already assists surgeons in a wide range of applications. Robots can not only assist in performing a surgery but can also access specific body parts with more precision than a human surgeon would. The biggest benefits of robot-assisted surgeries are minimized surgery time, reduced risks of blood loss, and shorter recovery time for the patients.
Expert Opinion
AI is transformative in healthcare, significantly enhancing patient outcomes through various applications. One of the primary uses of Artificial Intelligence is in diagnostics, particularly through computer vision, which enables the analysis of medical images such as X-rays, MRIs, and CT scans. AI algorithms can detect abnormalities that human eyes may overlook, such as early signs of cancer, fractures, or other medical conditions. This enhanced diagnostic capability helps healthcare providers make more accurate diagnoses and develop more effective treatment plans, saving time and improving decision-making in uncertain cases.
AI has also streamlined healthcare operations in areas like dermatology, where it can analyze skin lesion images and provide diagnoses based on visual patterns. This assists dermatologists in offering more precise diagnoses and personalized treatment plans. Additionally, Artificial Intelligence is being used to manage patient records, optimize scheduling, and allocate resources more effectively, leading to increased efficiency and cost savings in healthcare systems.
Cost reduction is another significant benefit of AI in healthcare. AI-powered tools can reduce the duration of preliminary diagnostics by analyzing symptoms and medical images to suggest further steps, such as whether a patient needs to see a doctor. This form of AI-based triage prevents unnecessary visits, saving patients and healthcare providers time and money while ensuring that those in need receive care promptly.
Looking to the future, Artificial Intelligence has the potential to drive innovations in personalized medicine, predictive analytics, and remote monitoring. AI could tailor treatments to individual patients based on their genetics, lifestyle, and medical history, leading to more effective outcomes. Predictive analytics powered by AI can help identify high-risk patients and foresee complications before they occur. Furthermore, AI-driven remote monitoring devices can assist patients in managing chronic conditions from the comfort of their homes, reducing the need for frequent hospital visits.
Despite these promising advancements, integrating AI into healthcare faces several challenges. The healthcare industry is often slow to adopt new technologies due to data privacy, security, and liability concerns. Additionally, issues such as data quality, system interoperability, and regulatory compliance must be addressed. To successfully implement AI, healthcare providers need clear guidelines, as well as education and training for professionals to understand and embrace its benefits.
In the next 5-10 years, AI’s role in healthcare is expected to grow significantly. It will continue to enhance diagnostics, monitor patient outcomes, and optimize treatment plans, all while reducing costs and improving the quality of care. As Artificial Intelligence becomes more integrated into healthcare systems, staying informed about the latest advancements will be crucial for healthcare providers to maintain competitiveness in this rapidly evolving field.
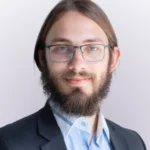
Steps to develop a machine learning solution for healthcare
If you consider implementing an ML solution in your organization or have an ML-based startup idea for the healthcare field, we highly recommend machine learning consulting services. Since the development of an ML solution is a highly complex and multifaceted process, it is important that you work with experienced professionals who will ensure that the delivered results precisely meet your current and future business goals. And to get an idea of what the development process looks like, below we list its core steps that are applicable to every ML project.
Identify the problem
While ML is an incredibly valuable tool, it will be pretty much useless if you don’t apply it to a specific problem. Hence, before assembling a team of data scientists and beginning the development process, it is vital that you outline your current issue, define how exactly the ML solution will help, and what KPIs will help monitor the progress.
Collect and prepare the data
To successfully train an ML model, you will need a (preferably) vast data set. So the next step in the ML model development process is data collection and preparation to later “feed” the data to the model.
There is a big variety of data sources in healthcare, including surveys, medical records, disease registries, or peer-reviewed literature. You can use only the data collected by your organization or combine it with existing and available reports. Just remember: the more data you have, the more accurate the results from the ML model will be.
Once your dataset is ready, you will need to prepare the data. Since the healhtcare data comes in many formats, it is often unstructured, meaning there can be missing values, inconsistencies, or similar issues. In order for the ML model to successfully analyze the data, it needs to be ‘cleaned”. The process includes removal of duplicate, corrupted, or inconsistent data from the dataset.
Train, validate, and test the ML model
Training and validation steps refer to actually teaching and fine-tuning the model. Model training happens during its development when the model “learns” what results it’s supposed to deliver. For that, a training dataset is used.
Next, you use a validation data set to see how well the model learned and whether any adjustments are needed. During this stage, it is still possible to optimize the model and implement changes. Finally, you test the model to get an unbiased evaluation of its performance. If you are satisfied with the results, the model is ready to be used.
Monitor and implement improvements
Once you deployed the model, it doesn’t mean the work stops. Since new data is generated on a regular basis, a model often needs to be retrained or fine-tuned to continue providing accurate results. Also make sure that the model implementation meets the defined KPIs and adjust the model accordingly.
The future of machine learning for healthcare
Today, the popularity of machine learning in healthcare is growing steadily though there are still several challenges that prevent its wider adoption. These challenges include lack of structured data, biased data, lack of expertise, and poor adoption strategies. However, healthcare professionals and data scientists are already working on these challenges, striving to make machine learning an integral part of the healthcare industry worldwide.
It is safe to assume that in the near future we will see machine learning in more and more healthcare facilities. This also means that Healthcare IT Services will grow in popularity since it is impossible to build a reliable and secure ML model without the external expertise of machine learning specialists. If you have any questions regarding the development of a custom ML model or simply want to assess its profitability and learn more about machine learning in healthcare, SoftTeco will gladly answer your questions and will provide you with more information upon request.
Comments