Table of Contents
In October 2021, Google introduced a brand new concept of AI architecture called Pathways which is intended to become a true breakthrough in AI development and in ML model training. And in April 2022, Google shared the performance and capabilities of PaLM – its large language model that has already set a shamelessly high bar for the existing LLMs. So what’s all the fuss about Pathways AI and where is it currently standing on the AI landscape? Let’s have a look.
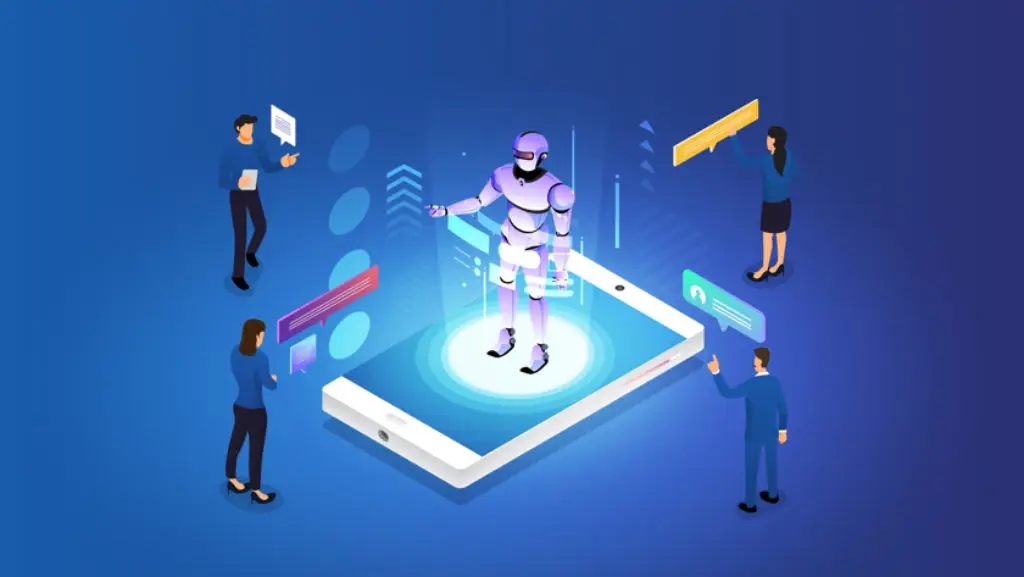
A quick overview of Pathways AI and the concept behind it
Pathways AI is intended to resolve the issues that current AI models are facing. To be more specific, Pathways will enable a single AI model to perform multiple tasks.
Now, what’s so unusual about it? To better understand the innovation behind the new AI architecture, we first need to understand how the classic AI model works.
What’s wrong with classic AI models?
While there is nothing critically wrong about the existing AI models since they deliver expected results, there are several issues that keep data scientists awake at night. They are:
- The inability of an AI model to solve several issues;
- Training of one model for one task only.
Let’s look at these issues in more detail.
When you build an AI model, you design it for a specific and single task and train this model for this specific task from the very beginning. Hence, a model is physically incapable of performing other tasks since it was designed for a single particular one.
Now, let’s compare this approach to the one that we, humans, use. When we learn certain information, we can apply it not for one but for many tasks and we can perform a task based on the skills that we already have. In other words, we are capable of multitasking.
What does it have to do with AI models and why is it important? The thing is, if we need several tasks to be done, we’ll need several models – and there is no need to say how expensive and time-consuming it may be. Just imagine how convenient it would be to have a single and powerful model instead. This brings us back to the Pathways.
The innovation behind Google Pathways AI
What Google aims to do is to create an AI architecture that would enable AI models to 1) perform thousands of tasks and 2) to use multiple senses to perceive information and deliver results. And if everything is more or less clear with multitasking, the use of multiple senses may sound confusing. Let us explain.
As Google puts it, Pathways will allow creating multimodal models that will encompass vision, auditory, and language for simultaneous understanding. That means, a model will be able to deliver a result whether hearing a word “flower”, seeing its image, or processing the word “flower”. Despite the way of receiving information, the output will be the same and that’s what makes Pathways AI so revolutionary. In addition to that, a model based on Pathways AI architecture will be able to use more abstract data for learning (i.e. the one that humans cannot interpret) and this will add to the accuracy of results.
PaLM: the first step towards making Pathways AI come to life
In April 2022, Google introduced the Pathways Language Model (PaLM) that uses 540 billion parameters and allows to train a single model across multiple TPU v4 Pods. As well, PaLM uses a standard Transformer architecture that all large language models use. Now, let’s pause for a moment and look at each point in more detail.
First, the number of parameters. Currently, PaLM is in the same league with its 540B parameters as OpenAI’s GPT-3 (175 billion), DeepMind’s Gopher and Chinchilla (280 billion) or GLaM and LaMDA by Google (with 1.2 trillion and 137 billion parameters, correspondingly). What we are trying to say here is that the bigger number of parameters does not equal better results and the accuracy really depends on the training efficiency.
Second, the use of TPU v4 Pods is worth your attention too. For those who don’t know, TPU stands for Tensor Processing Unit and is used specifically for AI models training. As you can guess from its name, TPU was designed by partially using the TensorFlow software, meaning, Google is the one standing behind the TPU design and development.
Now, what’s so special about the TPU v4? Each TPU v4 chip provides more than 2X the compute power of a TPU v3 chip. For PaLM model training, Google used two TPU v4 Pods. Now, a Pod is a network of 4,096 TPU v4 chips that are bound together with an ultra-fast interconnect (10X bandwidth per chip than a typical GPU-based training system). I’ll leave the efficiency of the final system to your imagination but here are the main things to remember: the use of TPU v4 Pods allows for significant decrease in latency and in network congestion.
Finally, the type of data used for PaLM training is also worth noting. The data used by Google included:
- Multilingual web pages: filtered in order to increase quality and limit content toxicity;
- English books;
- Multilingual articles from Wikipedia;
- English news articles;
- Multilingual social media conversations;
- Github source code.
So what results were delivered in the end? Here is what PaLM can already boast about in terms of tasks successfully resolved.
The breakthrough BIG-bench performance by PaLM
You’ve probably seen the famous “Imitation game” movie by Morten Tyldum that was released in 2014. The movie revolves around Alan Turing, the famous mathematician and computer scientist who invented the Turing machine and who came up with the “imitation game” test (later renamed as “Turing test”). If we refer to the official definition, the test evaluates “a machine’s ability to exhibit intelligent behavior equivalent to, or indistinguishable from, that of a human”.
What does it have to do with PaLM? The thing is, PaLM was tested on the BIG-bench which stands for Beyond the Imitation Game Benchmark. As you can guess, the company behind this benchmark is Google. The BIG-bench suite has over 150 language modelling tasks and all of them are aimed at determining how well an AI model can understand not only the language but the meaning of the phrase, cause and effect, etc. Examples of language modelling tasks that are included in BIG-bench are:
- conversational question answering;
- word sense disambiguation;
- grammar;
- dialogue system;
- common sense.
As for the PaLM achievements, they include correct guessing of the movie from an emoji, explanation of jokes, and common reasoning. What a time to be alive, indeed.
What’s the future of AI with Pathways?
The development of such AI architecture as Pathways will likely cause many questions, including ethical considerations, the future state of existing systems, and many others. But from the practical point of view, Pathways AI is a real breakthrough in terms of the use of computing power and the cost of building AI models. If we can build a single powerful model capable of performing several tasks, it may be much more financially rewarding than building and training several models.
While Google Pathways AI is still under development, we can only make theories about the potential use cases for such powerful models. So for now, let us sit back and observe Google working on one of the most interesting game-changers in the world of technology that scientists fifty years back only dreamed about.
Comments